일 | 월 | 화 | 수 | 목 | 금 | 토 |
---|---|---|---|---|---|---|
1 | 2 | 3 | 4 | 5 | ||
6 | 7 | 8 | 9 | 10 | 11 | 12 |
13 | 14 | 15 | 16 | 17 | 18 | 19 |
20 | 21 | 22 | 23 | 24 | 25 | 26 |
27 | 28 | 29 | 30 |
- sqs
- git book
- executor
- testing
- RDS
- SecretsManager
- lambda
- boto3
- dagrun
- TaskFlow
- authoring
- github actions
- Git
- Task
- kinesis
- Data Firehose
- pipeline
- ci/cd
- dynamic task mapping
- S3
- Scheduling
- credentials
- airflow
- AWS
- Operator
- XCOM
- celery
- Concept
- mysql
- DAG
- Today
- Total
목록Airflow (25)
CS
Airflow Best Practices Best Practices — Airflow Documentation airflow.apache.org 변수 및 connection mocking 메타데이터 DB 유지보수 Airflow 업그레이드 및 다운그레이드 변수 및 connection mocking 변수나 connection을 사용하는 코드를 테스트한다면, 테스트 시 그것들에 접근할 수 있어야 합니다. 그것들을 데이터베이스에 저장하고 코드를 실행할 때 읽을 수 있지만 그러면 추가적인 시간이 소요됩니다. 이를 개선하기 위해 파이썬의 unittest.mock을 사용하여 가짜 객체를 만들 수 있습니다. Airflow는 환경 변수에서도 변수와 connection을 확인하므로 mock.patch.dict로 os.en..
Airflow Best Practices Best Practices — Airflow Documentation airflow.apache.org DAG Loader 테스트 유닛 테스트 자가 테스트 환경 스테이징 예시 DAG Loader 테스트 python dag_file.py DAG 파일을 파이썬으로 실행하는 것만으로 설치되지 않은 의존성, 문법 오류 등이 있는지 확인할 수 있습니다. 물론 실행 환경이 실제 DAG를 처리할 Airflow scheduler가 속한 환경과 동일한 조건을 가져야 합니다. 또한 테스트는 DAG 로딩 최적화를 위해 사용할 수도 있습니다. Linux의 time 명령어를 통해 기존 실행 시간과 최적화 후 실행 시간을 비교할 수 있습니다. 캐시 효과를 고려하여 몇 번 실행한 후의 값을..
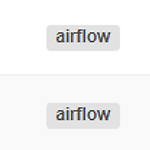
Airflow Dynamic DAG Generation Dynamic DAG Generation — Airflow Documentation airflow.apache.org 환경 변수를 통한 DAG 생성 embeded 메타데이터를 통한 DAG 생성 구조화된 데이터 파일을 통한 DAG 생성 동적 DAG의 등록 DAG 파싱 최적화 예시 For 반복문 등을 사용하면, 유사한 구조를 가진 여러 DAG를 DAG 하나하나마다 정의할 필요 없이 생성할 수 있습니다. 이를 동적 DAG 생성이라고 합니다. 이렇게 만들어지는 DAG들은 내부 task의 수가 기본적으로 같다는 점에서 동적 task 매핑과 구별됩니다. 환경 변수를 통한 DAG 생성 코드에서 변수를 사용하고 싶다면, top level에서는 Airflow 변수가..
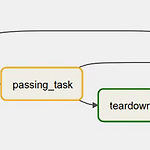
Airflow Best Practices Best Practices — Airflow Documentation airflow.apache.orgTask 작성 시 유의사항Task 제거Task 간 데이터 전달Top level 코드동적 DAG 생성DAG 변경 후 트리거Trigger rule과 Watcher pattern예외 AirflowClusterPolicySkipDag를 클러스터 정책에서 사용하여 특정 DAG 제외 Task 작성 시 유의사항 Airflow의 task는 데이터베이스에서의 트랜잭션과 동일하게 취급해야 합니다. 이는 task가 불완전한 결과를 도출하지 말아야 한다는 것입니다. 하나의 task는 말 그대로 하나의 작업이 되어야 합니다. Airflow는 task가 실패할 경우 재시도합니다. 따라서 ..
Airflow Logging for Tasks Logging for Tasks — Airflow Documentation airflow.apache.org Astronomer Airflow logging Airflow logging | Astronomer Documentation An introduction to Airflow logging. docs.astronomer.io 기본 개념 구성 로그 출력 코드로 task 로그 기록 worker와 triggerer에서 로그 전송 외부 저장소에 로그 전송 Logging Airflow는 기본적으로 task 로그를 남기며, 이를 Airflow UI에서 각 task별로 확인할 수 있습니다. Airflow는 기본적으로 task 로그를 파일로 남기는 FileTaskHa..
Airflow Timetables Timetables — Airflow Documentation airflow.apache.org Astronomer DAG scheduling and timetables in Airflow DAG scheduling and timetables in Airflow | Astronomer Documentation One of the fundamental features of Apache Airflow is the ability to schedule jobs. Historically, Airflow users scheduled their DAGs by specifying a schedule with a cron expression, a timedelta object, or a..
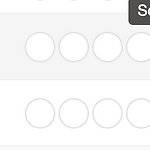
Airflow Data-aware scheduling Data-aware scheduling — Airflow Documentation airflow.apache.orgAstronomer Datasets and data-aware scheduling in Airflow Datasets and data-aware scheduling in Airflow | Astronomer DocumentationUsing datasets to implement DAG dependencies and scheduling in Airflow.docs.astronomer.ioDataset?DAG에서 dataset 사용하기여러 dataset을 함께 사용하기UI에서의 datasetDAG 예시데이터에 의한 스케줄링DAG를 시간에 기..
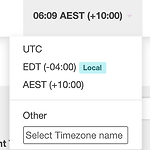
Airflow Time Zones Time Zones — Airflow Documentation airflow.apache.org Pendulum Documentation | Pendulum - Python datetimes made easy Installation Installing pendulum is quite simple: or, if you are using poetry: Introduction Pendulum is a Python package to ease datetimes manipulation. It provides classes that are drop-in replacements for the native ones (they inherit from them). Special pendu..